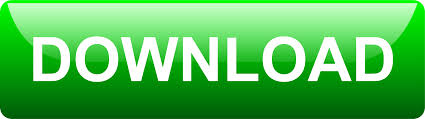
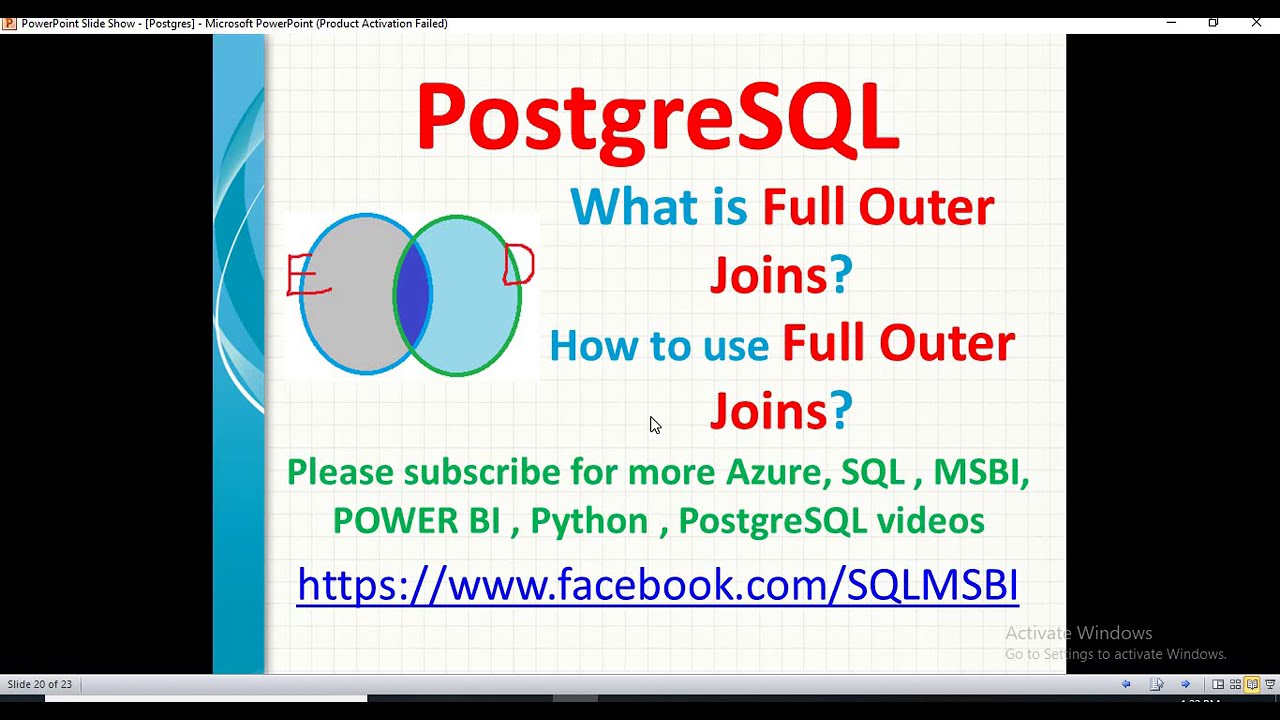
#POSTGRES ANTI JOIN FULL#
It turns out there is a pretty easy way to solve this - FULL OUTER JOIN ON FALSE. And even if you could, wouldn’t you want to do this in a more efficient way? Is there one? The section wherein the reader learns of the point of the article This would be prohibitive to run on virtually any data warehouse.

For context, if each row was 100 bytes, that would be 100’s of petabytes of data. This grows geometrically, so that if you wanted to pull a complete funnel, you might have 10,000 (pageviews) x 1,000 (add-to-carts) x 100 (orders) x 10 (upsells) = 10billion rows per product, which you probably have hundreds of, so your intermediate result set is trillions of rows. If we had higher cardinality relationships, for example, if you counted pageview events and add-to-cart events by product to calculate a click-through rate, you might easily have 10,000 (pageviews) x 1,000 (add-to-carts) = 10^7 rows per product in your intermediate result set. If you look at the Acme account in the above dataset, you’ll notice that there are 2 (products) x 3 (managers) = 6 rows. Problem 3 - Geometric growth of result set: Or, the elephant in the room. This would return the right number when grouping by accounts, but would return incorrect numbers when grouping by managers or products. To provide an example where this is particularly dangerous, consider if you had a key metric for accounts that was the ratio of products to managers. In non-trivial datasets, users can often be misled by this or, what is nearly as bad, can realize that the data is non-sensical and lose confidence in the data. However, this relationship and this measure are rarely intended by the LookML developer or end user. And, in this context, the count measures do make sense - they are the count of these relationships. In fact the rows of the table do represent a relationship - in our example, it enumerates pairs of products and managers that are related via an account. This is a more subtle problem than #1, though it does come up frequently. But what does that result set mean? On any given row, you have a product and a manager that are not actually related, but the table suggests they are. Problem 2 - Unintended relationships in table: If we do not choose to group to the account level, but instead select dimensions on account name, product name, and manager name, we would get precisely the above rows in our final result set. As already discussed, Looker automatically applies Symmetric Aggregates when necessary and gets us the right answers. Problem 1 - Measures/aggregates and double counting: As you can see from our example result set, aggregates would be wrong on all three of the tables, whether a sum of employees (we would expect 200, but would get 600), a count of products (we would expect 3 but get 7), or a count of managers (we would expect 4 but get 7). But double-counting is not the only problem… Specifying the problem(s)Īlthough we simply talk about “fan-out”, in truth, it’s not a singular problem, but rather 3 closely related problems. This Looker feature detects when there may be fan-out (via one_to_many declarations in your joins) and automatically applies distinct counts, and (rather sophisticated) sums of rows having distinct id’s, and so forth, in order to prevent double counting.

If you’ve been working with Looker for long, you’ll probably know about Symmetric Aggregates. You can tell already from all the repeated 80’s that you may run into problems with double-counting… But wait! Symmetric Aggregates! Here is the result of that fan-out, omitting duplicate or id columns for legibility: This animation shows how that result set is built, and we can see the fan-out in action: LEFT JOIN managers ON managers.account=accounts.idīefore we fill in the rest of the query, let’s visualize the result set before any grouping or aggregation happens. LEFT JOIN products ON products.account=accounts.id Assuming you had used the above explore definition, Looker would produce a query of the form. Let’s say a business user now wants to get counts of products and of managers by account. We informally call this situation “fan-out”. You probably already know that in SQL, this can lead to some unexpected results. Something along the lines of: explore accounts, left join products (1:M on accounts), left join managers (1:M on accounts). It feels very natural to write SQL like FROM accounts LEFT JOIN products or like FROM accounts LEFT JOIN managers, so many LookML developers default to writing an explore this way too. (For the time being, we’ll show de-normalized account names to make the examples easier to read) Let’s imagine we have the following three tables. Note: Although everything demonstrated in here is basic SQL, there are notable dialect considerations.
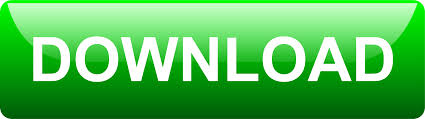